- Inventory management and forecasting: new uses
- How to calculate the assortment of products in store?
- Machine learning at the service of stock forecasting
The right product, at the right price, in the right place: this sentence alone sums up the problems linked to stock management. Which product does a customer buy? When does he buy it? Why this one and not another? How often? Verteego invites you to discover how to forecast and optimize stocks thanks to machine learning.
Inventory management and forecasting: new uses
Inventory management: a major challenge for companies
Inventory management refers to the management of a company's flow of goods, enabling the supply of products to meet customer demand. An optimal management of the latter can become a definite competitive advantage. In fact, managing transportation, product location, shelf or reserve supply, will allow us to respond quickly and efficiently to customer demand. In-store inventory management is influenced by customers' product choices and these behaviors often undergo changes that can be brutal. These uncertainties in inventory management must take into account the new calculation parameters that make it difficult for an individual to predict. This is why new stock management models integrate machine learning and take advantage of its data processing power.
Stock forecasting limited by the technique
Concerning the forecasting of stocks at the point of sale, several methods are possible. We are not going to detail the mathematical formulas here, but rather give a state of the art of the different techniques that can help in stock forecasting.
To carry out its calculations, one can of course use Excel and its many functions. This tool has the advantage of being accessible to everyone from a computer. However, it quickly becomes complicated to process and understand the data as they multiply. An Excel file with thousands of rows and hundreds of columns will only be useful if the views are filtered, to the detriment of its readability.
For companies that are adept at turnkey solutions, some ERPs specialize in inventory management, which makes it possible to delegate part of the task to software on a cloud or locally. Once again, it is enough if the database is too large for the solution to reach its limits. Another major issue is also the difficulty of these tools to update forecasting results in real time.
Today, there is software specialized in data analysis, but not all companies necessarily need analysis software to improve their sales forecasts, and therefore their stocks. It is then preferable to understand how customers act when they go to a point of sale, how they choose the products that will make up their shopping cart in order to anticipate the stocks necessary to satisfy their customers.
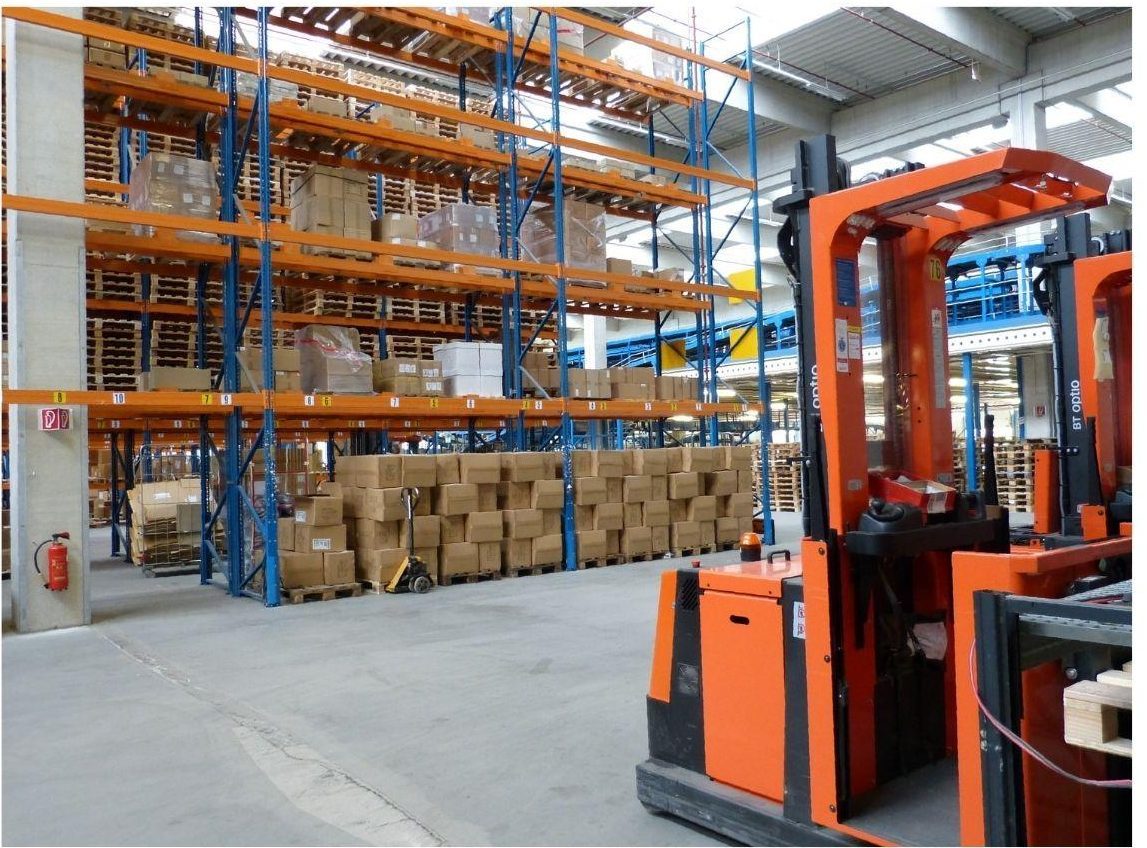
How to calculate the assortment of products in store?
Understanding your customers' buying motivations
Anticipating customer behavior through an analysis of internal company data such as sales receipts, for example, is undoubtedly the first step towards greater precision in inventory management aligned with the purchasing motivations of its customers.
In the case of a hypermarket, for example, the manual analysis of millions of sales receipts makes it more complex to provide relevant results in order to predict behavior. Even if these receipts indicate which customers buy which products, for what amount, at what period, and according to which promotions, this makes the work of the marketing and sales teams very time-consuming.
The analysis of the sales receipts will reveal recurring patterns in customer behavior. Thanks to these, it will be possible to identify the products that triggered the purchase, those for which the customers go to the point of sale or drive. If these products are not available, the act of purchase will not take place and the rest of the basket will be lost.
The analysis of sales receipts and the qualification of customer behavior will gather information on the most popular products, those that generate the most sales, and the timing of purchases. In optimizing inventory management, selecting the items to be put on sale is not always enough, so items must be prioritized. This can be done by respecting a limited number of items, by choosing the items that generate the most sales, or by prioritizing according to the choice of customers in a certain type of store.
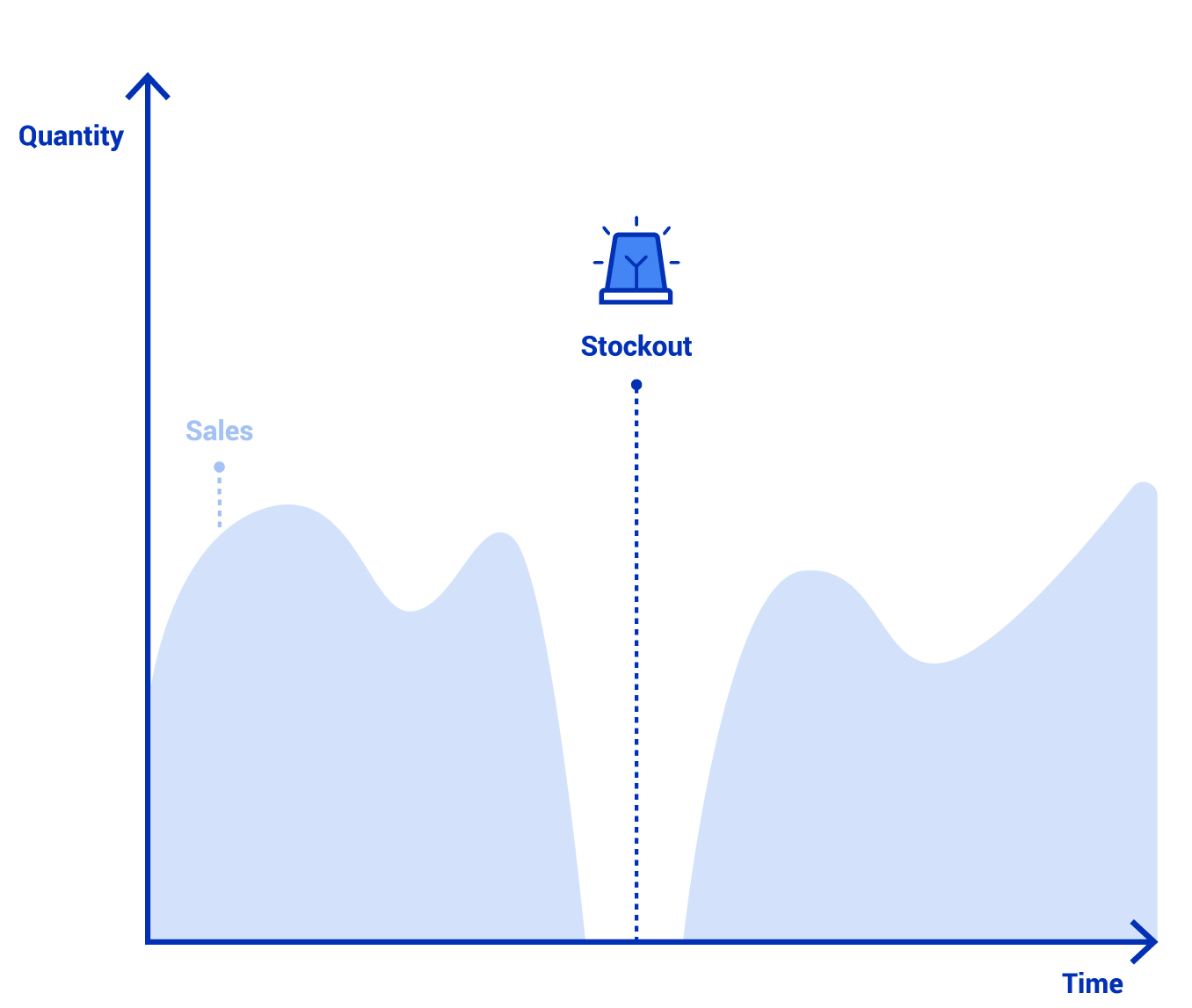
How much stock should be planned for each item?
If the analysis of customer behavior allows us to identify the items for which the consumer moves, one question still remains unanswered: how much stock to plan for each item offered for sale at the point of sale? How to optimise stock on the shelf? To answer this question, the classic analysis of sales receipts is not enough, it is also necessary to take into account internal and external factors. For example, a change in the amount of the average wage will directly impact the purchasing power of households, which would then change their purchasing habits. All this information represents an immense amount of data that cannot be analyzed by an individual. It is then a matter of calling on intelligence and also on machine learning.
Machine learning at the service of stock forecasting
Attention to customers and their behavior teaches us the importance of product availability on the shelf. If demand is too low, the point of sale will have a surplus of stock, which represents a shortfall in terms of turnover. If, on the other hand, demand is too high in relation to product availability, the item will be out of stock, reducing the number of items in the customer's final basket and creating a disastrous customer experience.
Optimizing stock forecasting with machine learning has many advantages:
- Considerable increase in sales efficiency due to better product availability.
- Improvement of the safety stock thanks to more accurate forecasting of replenishments on slow-moving items.
- Better understanding of "patterns" enabling correlation of data that do not appear to be related to each other, thanks to increased computing speed.
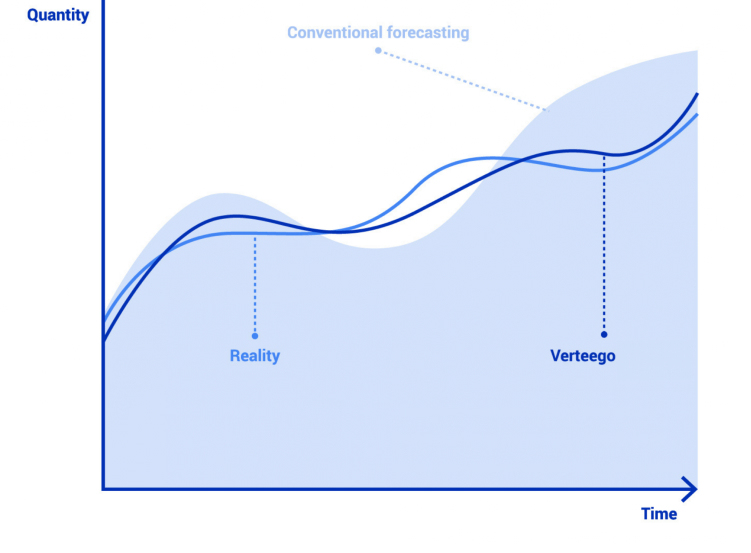
Stock forecasting and machine learning: the 4 key steps
Here are the four steps to implement a machine learning solution to optimize stock forecasting:
1 - Collect internal and external data
In order to train the prediction models, they need data to be analyzed. Internal and external data must then be collected. For example, a hypermarket will collect data on products, types of point of sale, prices or promotions. This data can then be supplemented with information on the weather, seasonality, economic factors or information on the competition.
2 - Define your business objective
This information is used to meet a specific objective defined by the point of sale. This can be the optimization of stocks but can also be the answer to the demand of its customers. Each business objective is translated into a mathematical model. At Verteego, we have built several customizable models in order to respond precisely to the initial objective.
3 - Training the models
Afterwards, it will be a matter of training the models. To do this, the data sets are integrated into the machine learning algorithms to enable them to begin the machine learning phase. It is these same models that we will be able to interrogate later on to generate predictions concerning customer behavior and, therefore, inventory management.
4 - Analyze the results
In order to visualize the results, several data visualization tools can be used. These include Google Data Studio or Verteego platform, which allows a clear and precise formatting of the data. Finally, each prediction is automatically reprocessed according to business operational issues. As an example: when a prediction is made to supply 3 quantities on a SKU packaged by 4, if the business objective is to reduce storage risks as much as possible, the prediction will recommend to store 2 quantities. On the other hand, if the business objective is to increase turnover as much as possible, the forecast will recommend to stock 4 quantities.
To conclude, machine learning facilitates the management of complex and exponential data. Thanks to an in-depth knowledge of product information and consumer habits, stock forecasting is much more finely optimized. The points of sale that use machine learning manage to reduce inventory costs, transport costs and waste, while increasing the productivity of the teams.
Do you want to optimize your stocks thanks to machine learning? Ask for a demo of our Verteego platform now.